Tellistic's case study of Using Power BI to Tackle Fraud Detection
- Tellistic
- Mar 26
- 3 min read
Updated: Apr 1
How Tax Authorities and Financial Institutions Are Using Power BI to Tackle Fraud Detection
Fraudulent activities, particularly Value Added Tax (VAT) fraud, pose significant challenges to tax authorities worldwide. The sheer scale of transactional data, combined with sophisticated fraud schemes, has made manual detection virtually impossible. Recognizing this, Tellistic Technology Services partnered with tax authorities from over three countries to revolutionize fraud detection by leveraging advanced statistical and machine learning models alongside Power BI analytics. This innovation bridges the gap between academia and operational data, empowering business intelligence analysts to detect suspicious behavior with unprecedented precision.
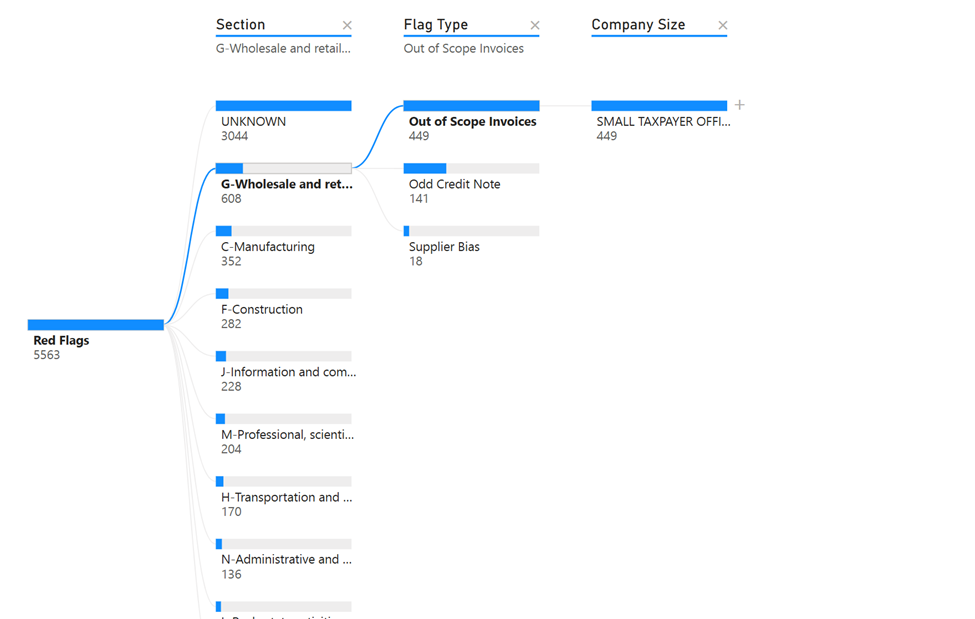
One prominent case involved a national tax authority aiming to improve its ability to identify non-individual taxpayers engaging in suspicious VAT fraud schemes. VAT returns, filed monthly, generate millions of transactions, creating immense challenges for analysts. Among the most complex schemes to detect is the "missing trader" fraud, where a network of accomplice taxpayers manipulates invoices to defraud the tax system. Conventional methods often fail to flag such schemes promptly, allowing fraud to persist unchecked.
Tellistic Technology’s approach was transformative. At the core of the solution was the integration of machine learning models such as Self-Organizing Maps, Basket Analysis, White Forest Algorithms, and Red Flag Theory. These models, long used in academic research, were adapted to handle operational taxpayer data, offering actionable insights. A comprehensive dashboard was developed using Power BI to bring these insights to life. Every taxpayer was assigned a risk score on a monthly basis, informed by the models’ analysis of patterns and anomalies in VAT returns.
The Self-Organizing Maps flagged anomalies in transaction patterns, while Basket Analysis tracked unusual co-occurrences of transaction types among networks of taxpayers. The White Forest Algorithm, a variation of ensemble machine learning, was particularly effective at identifying outliers and high-risk taxpayers amidst millions of data points. Combined with the Red Flag Theory, which focuses on predefined indicators of fraud, the system could not only assign risk scores but also identify specific fraud schemes associated with each taxpayer.
The dashboard delivered on Power BI offered unparalleled functionality. Taxpayers were ranked by risk scores, and potential fraud schemes were highlighted. With Power BI’s drill-down tools, analysts could explore individual entities in detail, examining transaction histories and interconnected networks of accomplices. This allowed them to make informed recommendations about whether a taxpayer warranted further investigation or if flagged patterns were false positives. The feedback loop didn’t stop there—the models were designed to learn continuously from analysts’ inputs, adapting to evolving fraud tactics.
This intervention had a far-reaching impact. Analysts could now identify high-risk taxpayers and widespread schemes, such as missing trader fraud, in near real-time. It enabled a shift from reactive responses to proactive fraud prevention. Additionally, the dashboards facilitated resource prioritization, focusing investigative efforts on the most severe cases.
By harnessing machine learning and Power BI, Tellistic Technology Services has transformed fraud detection into a data-driven science. The adaptability of the system ensures it remains effective against the ever-changing landscape of VAT fraud. As more tax authorities adopt these tools, the potential to safeguard national revenues and maintain trust in tax systems has never been greater. This case demonstrates the power of technology in tackling fraud at scale and highlights the pivotal role that innovation continues to play in public finance.
Comments